Acoustic Classification by Sonar
Disparate approach successful in cost-effective mapping
A breakthrough in signal conditioning has lead to significant improvements in single-beam and swath classification. With modern computers, this allows cost-effective bottom classification across multiple sonar platforms. The common dataset generated as a result of the highly successful shallow-survey conferences sponsored by the University of New Hampshire Ocean Mapping Group and the Defence Scientific and Technical Organisation of Australia is an ideal proving ground for classifying the same seabed using multiple platforms. The results of processing and classifying a common dataset are presented in this article.
Acoustic seabed classification is in wide use for applications from habitat mapping to mine countermeasures. Two key requirements are processing efficiency and reliable results. The QTC family of acoustic classification software for single-beam classification and for swath mapping was used to classify data from Portsmouth Harbour. The consistency of the classification approach across platforms allows the user interested in particular sites to work with a wider range of acoustic data for map making. The benefits of such processing and the consistency of classification results are focused on here.
Acoustic Classification
Geological and biological diversity of a seabed or riverbed is captured in sonar echoes from both sounders and imaging sonar. QTC classification products are designed to prepare echo data, highlight features and present data for mapping. Specifically, the software analyses the amplitude, shape and/or statistical nature of echoes as time series or as images. These signal components are determined mainly by sea-floor roughness, impedance mismatch between water and sediment and reverberation of the substrate. The resulting classification maps of acoustic similarity have many practical uses.
Sonar
Three surveys by very different sonar systems were found to give similar classifications.
- A Suzuki ES2025 colour fishing echo-sounder operating at 200 and at 50kHz produced echoes that were captured and digitised by a QTC VIEW Series V echo acquisition system. The echo time series were classified in QTC IMPACT software
- A Reson Seabat 8101 multibeam system operating at 240kHz produced bathymetry and images that were recorded in XTF. These images and supporting data were classified in QTC MULTIVIEW software
- A Klein 5500 sidescan system operating at 455kHz produced images that were recorded in XTF. These images were classified in QTC SIDEVIEW software
Classification Process
QTC classification systems all start with extensive quality control found to be absolutely essential for consistent classes free from artefacts. The raw material for generating features is stacks of echo time series and matrices of backscatter amplitudes from rectangular sub-images, for echoes and images respectively. The algorithms that generate features are distinct for the two types of data. For echoes the features capture shape and spectral character; with images the features express backscatter amplitudes and textures. A set of features from one stack or from one patch of image is called a Full Feature Vector (FFV). Once FFVs exist, the echo-based and image-based processes are the same: PCA and clustering. Principal-Components Analysis (PCA) gives three combinations of features that capture almost all survey variability; these three variables are called Q coordinates and are plotted in Q space. The final step, clustering, segments the acoustic data into classes that are acoustically homogenous in a statistically valid and useful way. Each rectangular sub-image gave a FFV and thus a point in three-dimensional Q space after PCA. Each stack of five echo traces also gave a FFV. Figure 1 shows how the classification processes merge after production of FFVs. Each dataset was split to six clusters, unsupervised, using the standard iterative maximum-likelihood technique. Stopping at six clusters was indicated by diminishing returns in total score for further splits. The plots in Figure 2 show these classes, at the resolution of one point for each FFV.
Classes
The cluster process assigns a primary colour to each class as it is created, regardless of its position in Q-space. Relationships within Q-space become more readily apparent if the colours are changed by scaling the red content to the Q1 coordinate of the cluster centres, Q2 to green, and Q3 to blue. With these so-called 'similarity' colours, classes that are acoustically similar are assigned similar colours on geographic class maps, as in Figure 3. The other change between Figure 2 and 3 is categorical interpolation. Most interpolation routines operate only with continuous variables. With classes, the only meaningful interpolated result is an integer. The QTC routines for similarity colours and categorical interpolation as embodied in QTC Clams, which provides visualisation of maps, took us from Figure 2 to Figure 3. Table 1 compares the classes from each sonar system in this survey area. The olive-coloured class dominated in each case, occupying between 62 per cent and 72 per cent of the survey area. Geotechnical descriptions originated with the plot of grain sizes of 22 sites from the Common Data Set, shown in the lower right plot of Figure 2. As far as possible, descriptions from any or all the sample sites within each acoustic class are listed in Table 1. For most classes the descriptions agree well across sonar systems, as one would expect from the similarities of the plots in Figure 3 and because the boundaries of the appropriate acoustic classes match those of the obvious rocks and sand waves.
Discussion
The tidal flow in and out of Portsmouth Harbour occurs mainly in the central deeper region of the survey area and is responsible for the major sand waves in the south centre and the minor group to the Northwest. To the east of this central area the channel shallows. East of the rock outcroppings the current is less and the sediments are finer. Both image-derived datasets, and to some extent the sounder classes, show that this eastern region is sands and muddy sands, distinct from the gravel and sandy gravel class shown olive. On mosaics of the Reson and Klein images there was little if any visual difference between the areas classified olive and these eastern regions, yet the QTC classification processes showed consistent sediment differences.
Conclusion
Acquisition of acoustic data is often an expensive and time-consuming process. Physical sampling of the seabed to determine bottom type adds to the effort and cost and furthermore produces data only at the specific sample sites. Tools which add value to the acquisition process, or to the data so acquired, have significant value for the surveyor or researcher. It is in this context that acoustic seabed classification excels, turning swath classification backscatter data into useful information and enabling confident extrapolation of point sample data over wide areas of the seabed. At only incremental cost, a simple single-beam echosounder becomes a seabed-mapping tool; a multibeam system provides more than just bathymetry. The results presented above provide evidence that consistency can now be seen across multiple platforms despite differences in data types and acquisition technology embodied in these platforms.
Acknowledgement
These datasets form part of the Common Data Set collected for the Shallow Survey Conferences (www. ccom.unh.edu/shallow/shallow_commo. htm). This article is based on a paper presented at the latest conference.
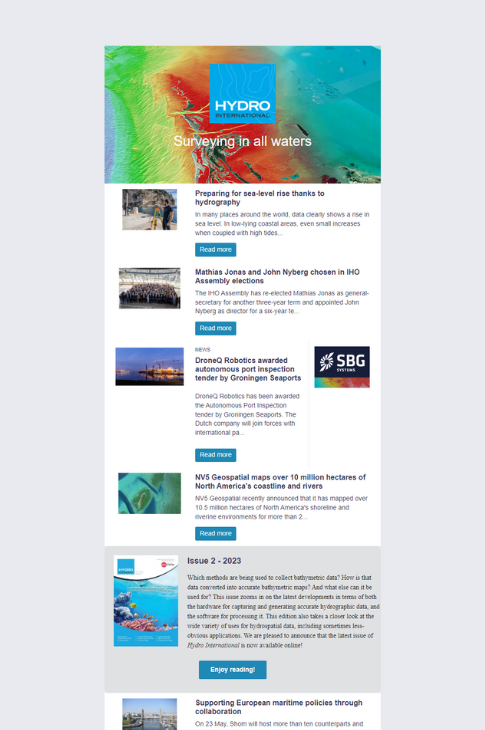
Value staying current with hydrography?
Stay on the map with our expertly curated newsletters.
We provide educational insights, industry updates, and inspiring stories from the world of hydrography to help you learn, grow, and navigate your field with confidence. Don't miss out - subscribe today and ensure you're always informed, educated, and inspired by the latest in hydrographic technology and research.
Choose your newsletter(s)